π‘ Educational Spotlight: π€ AI vs ML vs DL, π§ Critical Look at ChatGPT in Medicine, π Innovative Med AI Startups, and More! π
Educational Supplement to The 'Med AI' Capsule Newsletter π€π©Ίπ
Dear Med AI Enthusiast,
Welcome to this exclusive weekly feature, a carefully curated blend of valuable educational insights, complimenting your journey through The βMed AIβ Capsule - your essential weekly news digest π° on the dynamic intersection of artificial intelligence and other emerging technologies shaping the future of medicine. βοΈ
In todayβs supplement:
1 Concept Made Easy
1 Knowledge Resource
1 Research Deep Dive
Industry Showcase - 3 Innovative Startups/ Companies
GenAI Corner
Test Your Knowledge
Reading Time: 5-7 minutes
Concept Made Easy π¨βπ«
Artificial Intelligence (AI) vs Machine Learning (ML) vs Deep Learning (DL)
Artificial Intelligence (AI) is like giving computers or machines the ability to think and learn like humans. It helps them understand and solve problems on their own. π€
Machine Learning (ML) is a subset of AI that allows computers to learn from examples and experiences. It's like teaching a computer to recognize patterns in data and make predictions based on what it has learned. π
Deep Learning (DL) is a subset of Machine Learning that uses a more advanced learning methodology that is inspired by how our brain works. It uses artificial neural networks to learn and understand complex patterns. π§
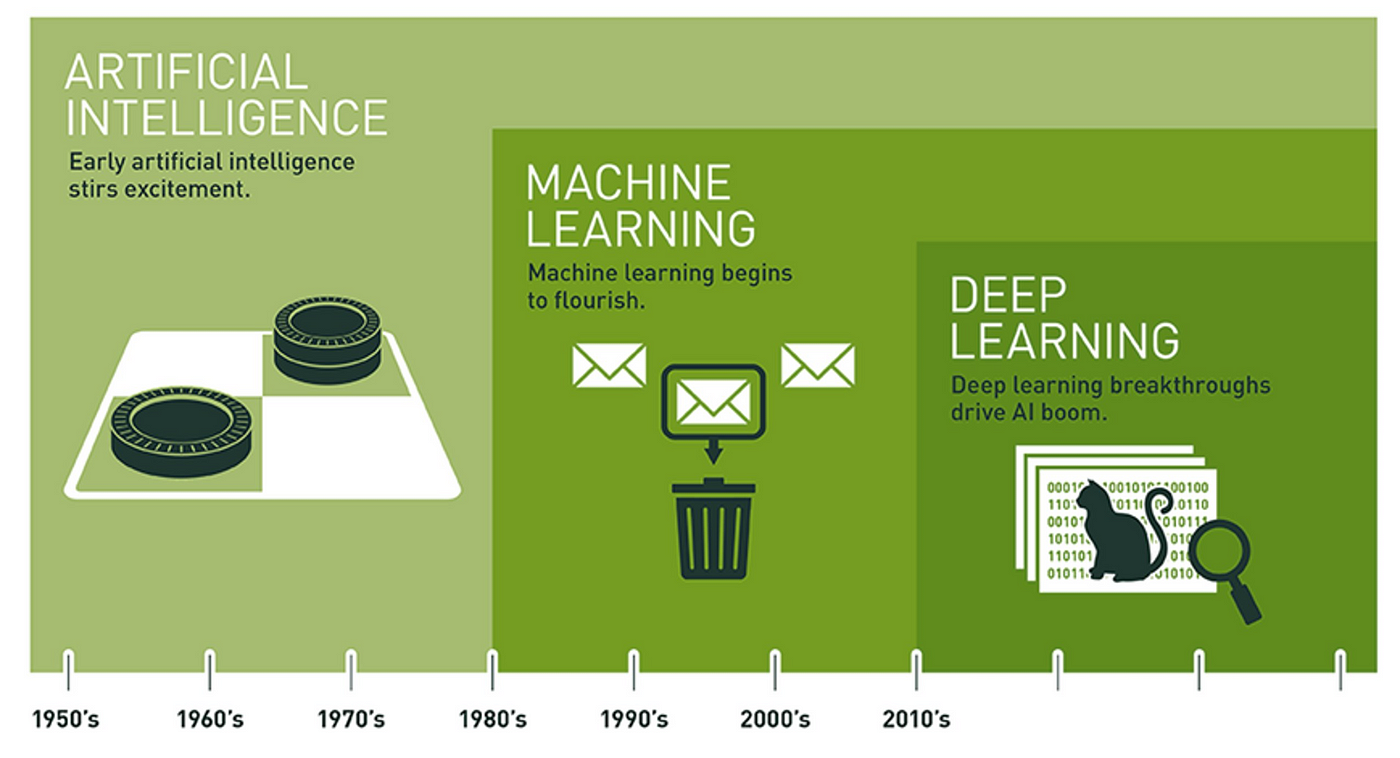
Here's a simplified timeline of how AI has evolved into ML and then DL:
Artificial Intelligence (AI) - 1950s to Present:
In the 1950s, scientists began exploring the concept of AI and developed early computer programs to perform tasks that required human-like intelligence. π§
Early AI systems focused on rule-based programming, where explicit instructions were given to computers to solve specific problems. πͺ
Examples include programs that played games like checkers and chess, where computers were programmed with rules to make strategic moves. βοΈ
Machine Learning (ML) - 1980s to Present:
In the 1980s, ML emerged as a subfield of AI, shifting the focus towards algorithms that could learn from data. π
ML algorithms were designed to analyze patterns and make predictions based on examples or experiences, without needing explicit programming for every scenario. π
ML found practical applications in various fields, including email spam filtering, recommendation systems, and predictive modeling. π©
Examples in healthcare include ML models that can predict disease outcomes or identify patterns in patient data for personalized treatment plans. π
Deep Learning (DL) - Late 1990s to Present:
DL is a subset of ML that emerged with the rise of powerful computers and the availability of large datasets. ποΈ
In the late 1990s, DL gained momentum with the development of deep neural networks, inspired by the structure of the human brain. π§
DL models consist of multiple layers of artificial neurons that can learn hierarchical representations of data.
DL has achieved remarkable success in tasks such as image and speech recognition. π£οΈ
In healthcare, DL has been used for medical imaging analysis, disease diagnosis, drug discovery, and genomics research. π©»
Over time, we progressed from rule-based AI systems to ML, where machines could learn from data, and then to DL, where deep neural networks enabled the understanding of complex patterns. π€
These advancements have expanded the possibilities of AI in various domains, including healthcare, where ML and DL models continue to show great potential for improving diagnostics, treatment, and patient care. π₯
So, in summary, AI is the concept of making machines smart, ML is a way for machines to learn from examples, and DL is a more advanced type of ML that can understand complex patterns. πΈοΈ
In healthcare, ML and DL can assist in diagnosing diseases, analyzing medical images, and making predictions based on patient data. π
Knowledge Resource π
Research Deep Dive π¬
Gravina AG, Pellegrino R, Cipullo M, Palladino G, Imperio G, Ventura A, Auletta S, Ciamarra P, Federico A. May ChatGPT be a tool producing medical information for common inflammatory bowel disease patients' questions? An evidence-controlled analysis. World J Gastroenterol. 2024 Jan 7;30(1):17-33. doi: 10.3748/wjg.v30.i1.17. PMID: 38293321; PMCID: PMC10823903.
This paper evaluates the potential of ChatGPT, a large language model (LLM) system, to provide medical information (MI) for common questions asked by patients with inflammatory bowel diseases (IBD). The study aims to understand if ChatGPT can be a reliable source of MI, addressing the accuracy, update frequency, and limitations of the information provided by the AI system.
Key Findings:
Potential and Limitations of ChatGPT: The analysis of ChatGPT's responses to IBD-related questions revealed that while the AI system shows some scientific reliability, it has notable limitations. These include a lack of detailed and up-to-date information and sometimes providing inaccurate information.
Specific Question Analysis: The study evaluated ChatGPT's responses to ten common questions from IBD patients. It found that while ChatGPT correctly addressed some topics, it fell short in providing nuanced and updated information, especially regarding the latest treatments and understanding of IBD.
Scientific Reliability: ChatGPT's responses were found to be scientifically plausible in some instances but lacking in others, particularly in terms of current medical knowledge and detailed guidance.
Conclusion: The study concludes that while ChatGPT demonstrates potential in providing MI for IBD patients, it requires further refinement and updating to ensure its reliability. It highlights the importance of aligning ChatGPT's outputs with the latest medical evidence from reliable databases. The authors suggest that ChatGPT should not yet be implemented as a standalone tool for patient inquiries and emphasize the critical role of healthcare professionals in interpreting and supplementing AI-generated information.
Limitations:
Lack of Real-Time Updates: ChatGPT's knowledge base is not updated in real-time, leading to potential gaps in the latest medical research and treatment options.
Generalized Responses: The AI system may not provide nuanced answers tailored to individual patient situations, which is crucial in medical contexts.
Absence of Clinical Judgment: ChatGPT lacks the ability to exercise clinical judgment, which is essential for providing personalized medical advice.
Dependence on Training Data: The quality of ChatGPT's responses is contingent on the diversity and reliability of its training data, which may not always cover all aspects of a complex field like IBD.
Overall, the paper underscores the importance of human oversight and the need for continued research and development in the use of AI tools like ChatGPT in healthcare settings, particularly for chronic and complex conditions like IBD.
Industry Showcase π¨βπ
Qure.ai - leverages AI to enhance healthcare accessibility and affordability. The company uses deep learning algorithms for rapid interpretation of radiology images, including chest X-rays, head CT scans, and more. Their technology also extends to public health solutions for COVID-19 and tuberculosis.
HealthifyMe - a digital health and wellness platform. The company's AI-based virtual assistant, Ria, assists users in multiple languages with fitness, nutrition, and health queries. Their app provides dietary recommendations and tracks calorie intake using AI.
Niramai Health Analytix - pioneering in the field of AI for breast cancer screening. Their product, Thermalytix, is a software-based medical device using high-resolution thermal sensing technology. This innovative approach offers an alternative to traditional mammography, aiming for early-stage detection of breast cancer. It's particularly beneficial in regular preventive health checkups and large-scale screenings in rural and semi-urban areas.
GenAI Corner π€
Tutorial: Using ChatGPT to Create Personalized Patient Education Materials
Step 1: Define the Medical Condition or Topic
Start by clearly identifying the medical condition or health topic you want to create educational material about. For instance, "Type 2 Diabetes."
Step 2: Outline Key Points to Cover
List the essential points you want to include in the material. This might include causes, symptoms, treatment options, lifestyle changes, etc.
Step 3: Craft a Detailed Prompt for ChatGPT
Write a prompt for ChatGPT that includes your topic, the key points, and any specific style or format requirements. For example:
"Create a patient-friendly guide on Type 2 Diabetes covering its causes, symptoms, treatment options, recommended lifestyle changes, and dietary advice. The guide should be easy to understand and suitable for patients newly diagnosed with Type 2 Diabetes."
Step 4: Refine the Output
Once ChatGPT provides the initial draft, review it for medical accuracy, clarity, and tone. If necessary, ask ChatGPT to make specific revisions, like simplifying medical jargon or adding more details on a certain aspect.
Step 5: Personalize and Finalize
Add any final personal touches that reflect your practice's approach or specific advice you give to your patients. Ensure that the material aligns with your professional standards and guidelines.
Test Your Knowledge π§©
Your feedback is crucial to me, as it helps me understand your interests and improve my offerings. I would appreciate it if you could take a few minutes to share your thoughts about what you've enjoyed and what you think I could do better - https://forms.gle/fUJZhjUBJe9ojySg6
Stay tuned for our upcoming editions as we explore the latest breakthroughs and dive deep into the transformative power of artificial intelligence and emerging technologies, shaping a healthier future. π
Warm regards,
